Introduction
Artificial intelligence forecast technology is revolutionizing how we predict financial trends. Businesses now leverage AI to gain deep insights, foresee market shifts, and optimize decision-making. Here’s a quick look at why AI forecasting stands out:
- Improved Accuracy: AI handles vast datasets and uncovers hidden patterns.
- Increased Efficiency: Automation speeds up data analysis and model training.
- Faster Decision-Making: Real-time insights keep forecasts current.
- Better Insights: Advanced techniques reveal connections missed by traditional methods.
- Competitive Advantage: AI helps businesses stay ahead by anticipating market changes.
At Russell's company, Profit Leap, we provide financial and strategic consulting, bookkeeping, and business intelligence services for small businesses, helping them harness AI for better financial forecasting.
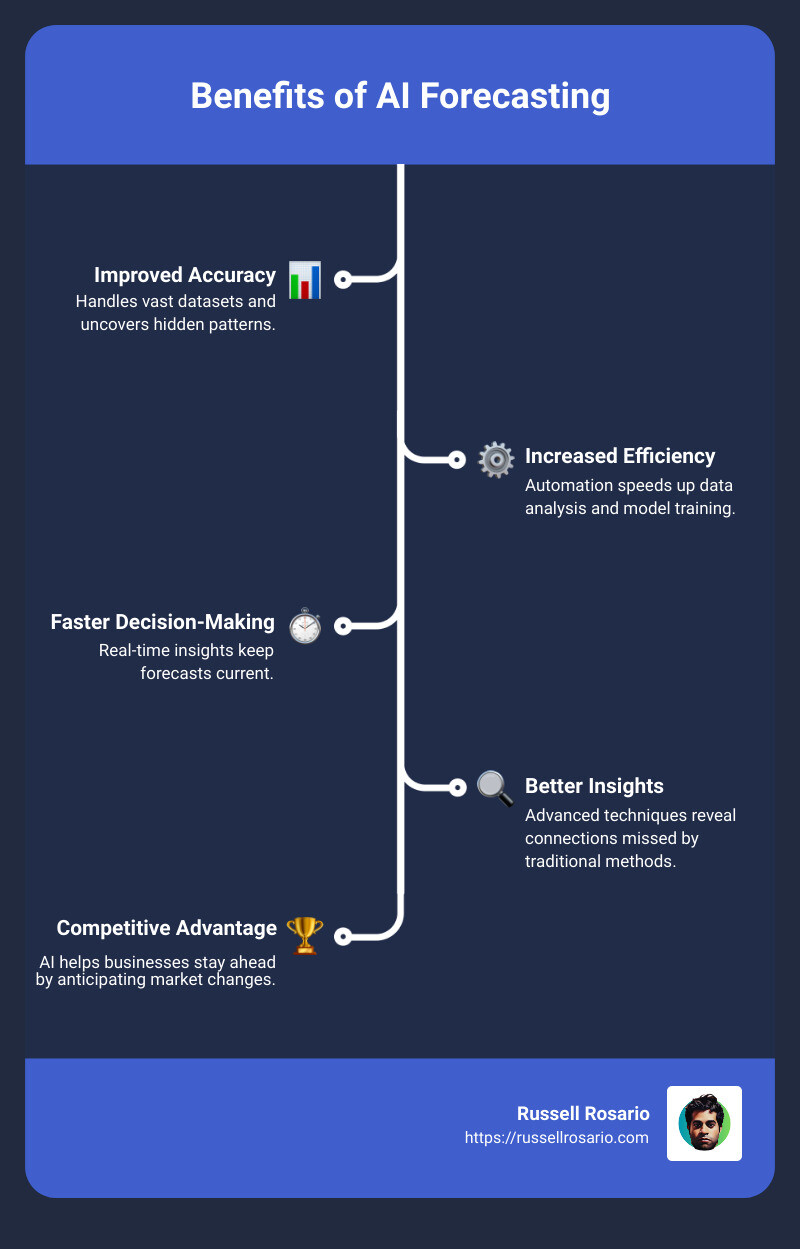
The Evolution of Forecasting Methods
Traditional Forecasting Methods
Traditional forecasting methods rely heavily on statistical techniques and historical data. These methods include simple linear regression, moving averages, and even more complex models like ARIMA (AutoRegressive Integrated Moving Average).
- Statistical Techniques: These methods use mathematical formulas to analyze historical data and predict future trends. Common techniques include mean, median, and standard deviation.
- Historical Data: Traditional methods often depend on past data to make future predictions. While this can be effective for stable environments, it struggles with dynamic and complex data.
However, these methods have several limitations:
- Inflexibility: Traditional techniques often fail to capture complex patterns and non-linear relationships in data.
- Human Bias: Predictions may be influenced by the analyst's biases, leading to inaccurate forecasts.
- Scalability Issues: Handling large datasets can be challenging, making it difficult to scale.
AI-Powered Forecasting
AI forecasting leverages machine learning and other advanced techniques to overcome these limitations. AI models can analyze vast amounts of complex data in real-time, offering several advantages:
- Machine Learning: This subset of AI involves training algorithms on historical data to recognize patterns and make predictions. Unlike traditional methods, machine learning can handle both structured and unstructured data.
- Complex Data: AI excels in managing intricate, non-linear relationships within data. It can process high-dimensional datasets that traditional techniques struggle with.
- Real-Time Forecasting: AI models can be configured for real-time analysis, providing up-to-date insights and enabling faster decision-making.
- Scalability: AI systems are designed to scale efficiently, accommodating growing datasets and increasing complexity.
For example, in website traffic forecasting, an AI model like ARIMA can identify trends and seasonality, helping businesses optimize their marketing strategies. In sales forecasting, AI models like ETS (Error, Trend, Seasonality) can analyze seasonal fluctuations and errors, providing valuable insights for inventory management and staff optimization.
In summary, while traditional forecasting methods have their place, AI-powered forecasting offers a more accurate, efficient, and scalable solution for modern businesses. This shift is crucial for staying competitive in a rapidly evolving market.
Next, let's explore the benefits of AI in financial forecasting and how it can transform your business operations.
Benefits of AI in Financial Forecasting
Improved Accuracy
One of the biggest advantages of using artificial intelligence forecast models is improved accuracy. AI can handle large datasets and recognize patterns that humans might miss. Traditional methods often struggle with non-linear relationships in data. AI, however, excels at identifying these complex patterns, leading to more precise predictions.
For example, AI models can predict stock market trends by analyzing vast amounts of historical and real-time data, detecting subtle patterns that influence stock prices.
Increased Efficiency
AI automates many aspects of the forecasting process, making it more efficient. Tasks like data preprocessing and model training can be time-consuming when done manually. AI handles these tasks quickly and accurately.
Imagine automating your entire financial forecasting process—from gathering data to generating reports. This frees up valuable time for your team to focus on strategic tasks instead of getting bogged down in data management.
Faster Decision-Making
In today's business environment, real-time insights are crucial. AI can provide up-to-date forecasts, enabling faster decision-making. When market conditions change, AI can quickly adapt, providing your business with the latest insights.
For instance, if a sudden market shift occurs, AI can analyze the new data in real-time and offer actionable insights, allowing your business to respond promptly.
Better Insights
AI can uncover hidden patterns in data that traditional methods might overlook. Advanced techniques like deep learning allow AI to analyze complex datasets, providing deeper insights into market trends and customer behavior.
Consider how AI can help a retail business. By analyzing customer purchase history and behavior, AI can predict future buying trends, helping the business optimize inventory and marketing strategies.
Competitive Advantage
Using AI in financial forecasting gives your business a competitive edge. AI can help you anticipate market shifts, allocate resources more effectively, and better understand customer needs. This proactive approach allows you to stay ahead of competitors.
For example, companies like Amazon and Google use AI to predict consumer demand and optimize their supply chains, maintaining their leadership positions in their respective markets.
By integrating AI forecasting into your business strategy, you can achieve greater accuracy, efficiency, and insight, ultimately driving better decision-making and gaining a competitive advantage.
Popular AI Forecasting Models & Techniques
When it comes to artificial intelligence forecast models, there are several popular techniques that businesses use to predict future trends. Each model has its strengths and is suited to different types of data and forecasting needs. Let's dive into some of the most widely used AI forecasting models.
ARIMA (AutoRegressive Integrated Moving Average)
ARIMA is a powerful model for handling time series data. It combines autoregressive (AR) and moving average (MA) components to capture trends and seasonality. This makes it ideal for forecasting website traffic or stock prices, where understanding past patterns is crucial for predicting future values.
Use case: A retail company might use ARIMA to forecast sales over the next quarter, helping them optimize inventory and staffing levels.
ETS (Error, Trend, Seasonality)
ETS stands for Error, Trend, and Seasonality. This model is great for sales forecasting as it breaks down time series data into these three components. It helps businesses understand underlying trends and seasonal fluctuations, making it easier to plan for peak shopping seasons or promotional events.
Use case: A retail store could use ETS to forecast holiday sales, ensuring they have enough stock and staff to meet demand.
STL (Seasonal-Trend Decomposition using LOESS)
STL is another time series forecasting technique that decomposes data into seasonal, trend, and residual components. It uses LOESS (a method for estimating nonlinear relationships) to provide a flexible and robust analysis.
Use case: A company might apply STL to forecast regional sales, helping them understand seasonal variations in customer behavior.
Regression Models
Regression models estimate relationships between a dependent variable and one or more independent variables. They are versatile and can be used for both time series and cross-sectional data.
Use case: Businesses can analyze the ROI of marketing campaigns by using regression models to identify which channels drive the most sales and leads.
Bayesian Networks
Bayesian networks are probabilistic models that represent a set of variables and their conditional dependencies. They are useful for tasks like spam filtering, order planning, and threat detection.
Use case: An e-commerce platform might use Bayesian networks to detect fraudulent transactions, improving security and customer trust.
Evolutionary Algorithms
Evolutionary algorithms mimic the process of natural selection to solve optimization problems. They use techniques like mutation and recombination to explore a wide range of potential solutions.
Use case: Manufacturers can use evolutionary algorithms to optimize production schedules, reducing costs and improving efficiency.
Deep Learning
Deep learning models are excellent at handling unstructured data and complex patterns. They are particularly useful for specialized processes like image and speech recognition, as well as understanding human behavior.
Use case: A financial services firm might use deep learning to analyze customer sentiment from social media, helping them tailor their services to meet client needs better.
These AI forecasting models offer a variety of approaches to predict financial trends, each suited to different types of data and business needs. By choosing the right model, businesses can gain deeper insights and make more informed decisions.
Next, we'll explore how AI forecasting is being applied across different industries, from manufacturing to healthcare.
AI Forecasting in Different Industries
Manufacturing
In manufacturing, AI forecasting is a game-changer. The global supply chain is incredibly complex, with numerous suppliers and variables. AI helps balance supplies and sales to achieve a sales equilibrium that maximizes profits. Without AI, managing and optimizing these intricate networks would be nearly impossible. For instance, AI can predict when to order raw materials to avoid shortages or excess inventory, saving both time and money.
Healthcare
Healthcare is rapidly adopting AI forecasting to improve patient outcomes. Traditional methods often suffer from human bias, but AI offers data-driven treatments that are more accurate. AI can analyze vast amounts of medical data to predict disease outbreaks or recommend personalized treatment plans, significantly improving the quality of living for patients. For example, AI algorithms can forecast flu seasons, helping hospitals prepare in advance.
Retail
Retailers use AI forecasting to manage inventory and adapt to seasonal trends. For a global retail management system, it’s crucial to forecast when to swap inventory from summer to fall for different hemispheres. Imagine sending heavy winter coats to Australia just as temperatures begin to rise—AI helps avoid such costly mistakes. By analyzing past sales data and current trends, retailers can ensure they have the right products at the right time, enhancing customer satisfaction and boosting sales.
Financial Services
Financial services have embraced AI for investment solutions and robo-advice. AI developments allow for customized investment plans that were once only available to high net worth clients. These systems dynamically manage finances to match individual goals, like saving for a mortgage. AI can also analyze vast amounts of financial data to offer real-time advice, making financial planning more accessible and efficient.
High Tech
In high tech industries, AI forecasting is essential for developing cutting-edge technologies. Human intuition often falls short when predicting novel trends, but AI provides reliable planning to navigate these uncertainties. Companies can use AI to forecast market demands, optimize R&D investments, and stay ahead of the competition. For example, AI can predict the next big tech trend, allowing companies to innovate and capture market share more effectively.
Next, we'll dive into the future of AI forecasting, exploring market growth projections, technological advancements, and industry adoption.
The Future of AI Forecasting
Market Growth Projections
The Artificial Intelligence (AI) market is on a rapid growth trajectory. From 2024 to 2030, the market value is expected to surge from approximately $214.6 billion to a staggering $1,339.1 billion. This represents a compound annual growth rate (CAGR) of 35.7%.
This explosive growth is driven by advancements in computational power and data availability, enabling more sophisticated AI algorithms and models. Governments, especially in regions like Asia Pacific, are heavily investing in AI R&D, creating a fertile ground for innovation.
Technological Advancements
Technological advancements are a key driver of AI forecasting's future. Novel AI architectures integrating neuro-symbolic approaches, quantum computing, and biologically-inspired models are pushing AI capabilities beyond current boundaries.
- AI Chips: Companies like Intel and IBM are developing specialized AI chipsets to handle complex computations more efficiently. These chips are crucial for real-time data processing and model training.
- Edge Computing: AI at the edge allows for real-time data analysis and decision-making without relying on cloud infrastructure. This is particularly useful for applications requiring low latency.
- Cloud Infrastructure: Cloud platforms like AWS and Google Cloud are continuously improving their AI capabilities, offering scalable solutions for businesses of all sizes.
Industry Adoption
The adoption of AI forecasting is widespread across various industries:
- Automation: AI is automating repetitive tasks, freeing up human resources for more strategic roles. For instance, in manufacturing, AI forecasts can optimize supply chains and production schedules.
- Optimization: AI helps in optimizing business processes by providing data-driven insights. Retailers use AI to manage inventory and predict seasonal trends accurately.
- Consumer-Facing Applications: AI-powered chatbots and virtual assistants are enhancing customer service by providing real-time support and personalized recommendations.
Regulatory Challenges
As AI becomes more integrated into business operations, several regulatory challenges need to be addressed:
- Data Privacy: With the increasing use of AI, data privacy has become a significant concern. Regulations like GDPR in Europe aim to protect consumer data, but businesses must navigate these laws carefully.
- Ethical Concerns: The ethical use of AI is under scrutiny. Issues like algorithmic bias and transparency in AI decision-making processes are critical for gaining consumer trust.
- Regulatory Acceptance: Governments are still catching up with the rapid pace of AI innovation. Regulatory frameworks need to evolve to ensure the safe and ethical deployment of AI technologies.
AI forecasting is transforming industries by providing accurate, real-time insights that drive better decision-making and efficiency. However, navigating the regulatory landscape and addressing ethical concerns will be crucial for sustainable growth.
Next, we'll explore popular AI forecasting models and techniques, including ARIMA, ETS, and deep learning.
Frequently Asked Questions about AI Forecasting
What is the forecast for AI technology?
AI technology is on a rapid growth trajectory. By 2030, the AI market is expected to surge from approximately USD 214.6 billion in 2024 to USD 1,339.1 billion. This growth is driven by advancements in computational power and data availability, which enable more sophisticated AI algorithms and models. The economic impact of AI is significant, with industries like manufacturing expected to gain $3.8 trillion by 2035 due to AI adoption.
What will AI be worth in 2025?
By 2025, the AI market value is projected to be substantial, with a continued high growth rate. The software segment, which includes discriminative and generative AI, will account for the largest market share. This segment's flexibility to adapt to various use cases makes it a critical driver of AI adoption across industries.
What is the prediction for AI in 2030?
Looking ahead to 2030, AI will not only grow in market size but also transform human capabilities and industries. Technological advancements like novel AI architectures, quantum computing, and integration with augmented reality (AR) and virtual reality (VR) will push AI capabilities beyond current boundaries. The industry impact will be profound, with AI optimizing urban planning, healthcare, and more, making cities smarter and more sustainable.
AI's future is bright, but it will require careful navigation of regulatory challenges and ethical concerns to ensure its benefits are realized responsibly.
Conclusion
As we've explored, the future of financial forecasting lies in the hands of artificial intelligence. The artificial intelligence forecast shows that AI will continue to revolutionize how businesses predict financial trends, improve efficiency, and make data-driven decisions.
At Russell Rosario, we are committed to helping businesses harness the power of AI forecasting. Our expertise in financial and strategic consulting, bookkeeping, and business intelligence services, provided through our partnership with Profit Leap, ensures that small businesses can leverage AI to enhance financial efficiency and profitability.
Russell Rosario, CPA, brings a wealth of experience to the table, guiding businesses through the complexities of AI integration. By utilizing advanced AI models and techniques, we enable companies to uncover hidden patterns, make faster decisions, and gain a competitive edge in their respective markets.
Incorporating AI into financial forecasting is not just about staying ahead; it's about transforming how we understand and respond to financial data. With AI, businesses can achieve data-driven decisions that lead to smarter growth strategies and better resource allocation.
To learn more about how AI can transform your financial forecasting, visit our Complete Guide to AI in Financial Forecasting.
Embrace the future of forecasting with AI and see your business thrive.